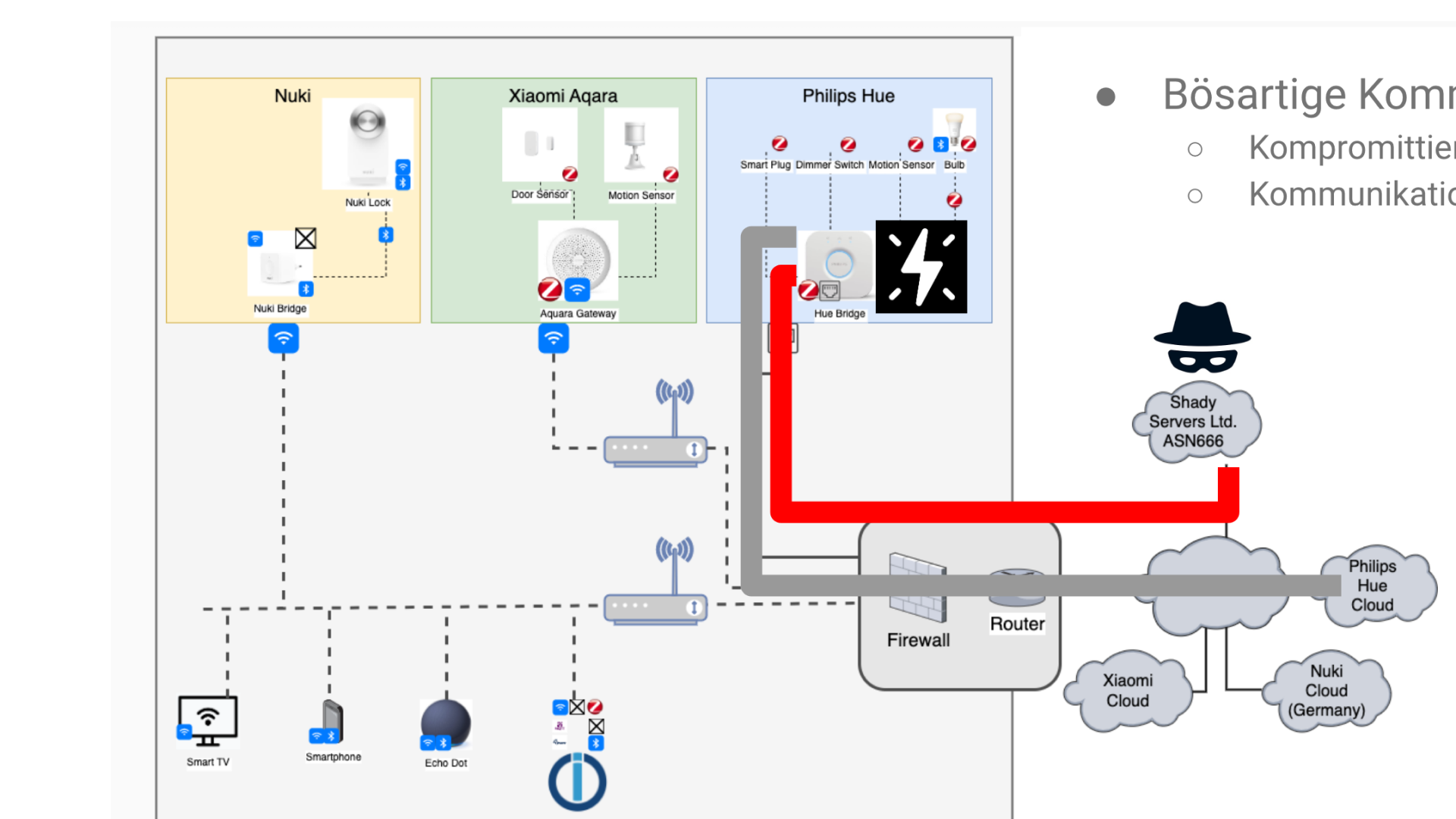
Motivation
Private households have a steadily growing number of network- and Internet-enabled smart home devices. Most of these are Internet of Things (IoT) devices, some of which are inadequately protected and do not allow for any retrofitting of protective measures. In addition, the increase in remote work means that the home network is increasingly becoming the focus of cybercriminals. Therefore, it is necessary to implement effective security systems in private homes as well, with protection and detection techniques that can detect and respond to cyberattacks at an early stage.
Threats to IoT and smart home devices have also become apparent, not least in parallel with the Russian Federation’s largely conventional military aggression against Ukraine, in which targeted malware attacks have destructively infected IoT devices such as Via SAT terminals, and rendered them inoperable. Corresponding collateral damage also ensured in Germany, for example, that wind turbines connected via satellite were no longer able to communicate. Similarly, infections with the Cyclops Blink malware targeted routers in order to be able to carry out large-scale overload attacks, even on uninvolved infected persons, against targets in Ukraine, presumably in order to provide tactical support for the conventional military conflict. Last but not least, there is also a direct threat to citizens in Germany through infections of IoT devices and a corresponding tapping of usable or personal data, possibly even extending to surveillance scenarios.
These threats are evolving rapidly and will be addressed in an agile manner by exploring detection and mitigation options, including the evaluation of machine learning (ML) techniques.
Goals and Approach
The project “AI-based and user-centric security solutions in the smart home (KISSHOME)” is dedicated to the protection of citizens’ home networks through the use of learning security systems. This includes the development of techniques and methods for privacy protection as well as for improved malware analysis, also under evaluation of suitable ML algorithms. In addition to researching ML-based methods for capturing and analyzing network traffic, we focus on the acceptance and usability of the self-learning security system. The focus is on the users with their attitudes, emotions and usage behavior. These criteria will be tested in acceptance and usability tests. In addition, barriers such as high prices for security solutions or the perception of a security system as a black box, where, for example, decisions made by the ML-based software cannot be traced, are to be avoided by making the project result available free of charge as an open-source solution in non-profit ecosystems.
Innovations and Perspectives
The user-friendly security system for protecting home networks, which is based on the implementation and use of novel ML algorithms, is intended to strengthen societal IT security. Since digital security in the private sphere is often neglected for reasons of usability or convenience, the user-centric approach is intended to minimize the barriers to using the technology. In this way, the project can contribute to the spread of secure technologies and to strengthening awareness of IT security in society.
The limitations of ML-based methods are also being deliberately explored. In an elaborate study on the analysis of functional limits in compiled code, the scientists found that a neural network, despite extensive optimization, performs worse than previous algorithmic methods. This work also shows that for certain problem domains in IT security, machine learning techniques use certain artifacts for classification. A scientific investigation of the datasets and the learning methods is even more important to gain robust insights.
The 3-year joint project KISSHOME is a cooperation of the working group around Prof. Dietrich of the Institute for Internet Security at the Westfälische Hochschule, the ARIC – Artificial Intelligence Center Hamburg e.V. and the Institute for Innovation Marketing at the Technical University of Hamburg (TUHH). It is funded by the German Federal Ministry of Research, Technology and Space (BMFTR) with a total of 1.8 million EUR under the funding code 16KIS1653
.
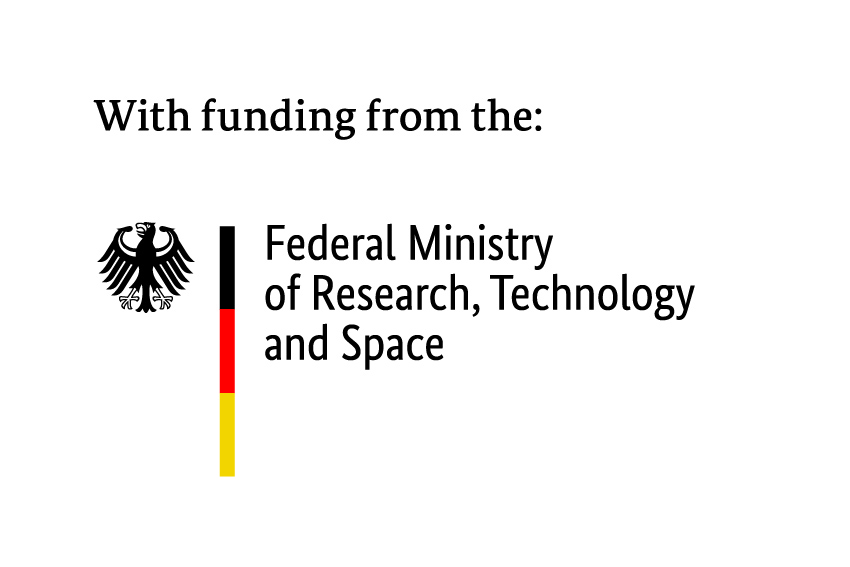
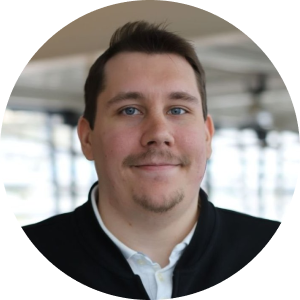
Daniel Großenbach
My research interests include computer security, malware analysis with focus on IoT malware and reverse engineering malware samples.
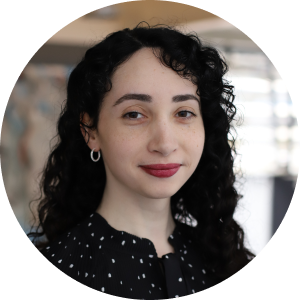
Sofie Telöken
My research interests include machine learning, data science, reverse engineering and malware analysis.
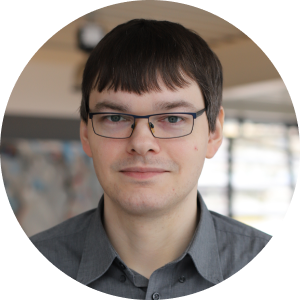
Gerhard Senkowski
My research interests include computer security, machine learning, and network intrusion detection.
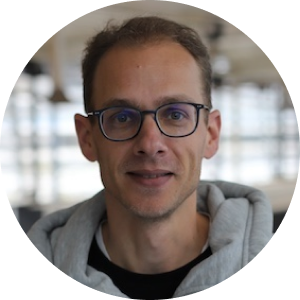
Prof. Dr. Christian Dietrich
My research interests include machine learning, computer security and threat hunting. Especially I use machine learning methods for virtual machine introspection based detection systems.